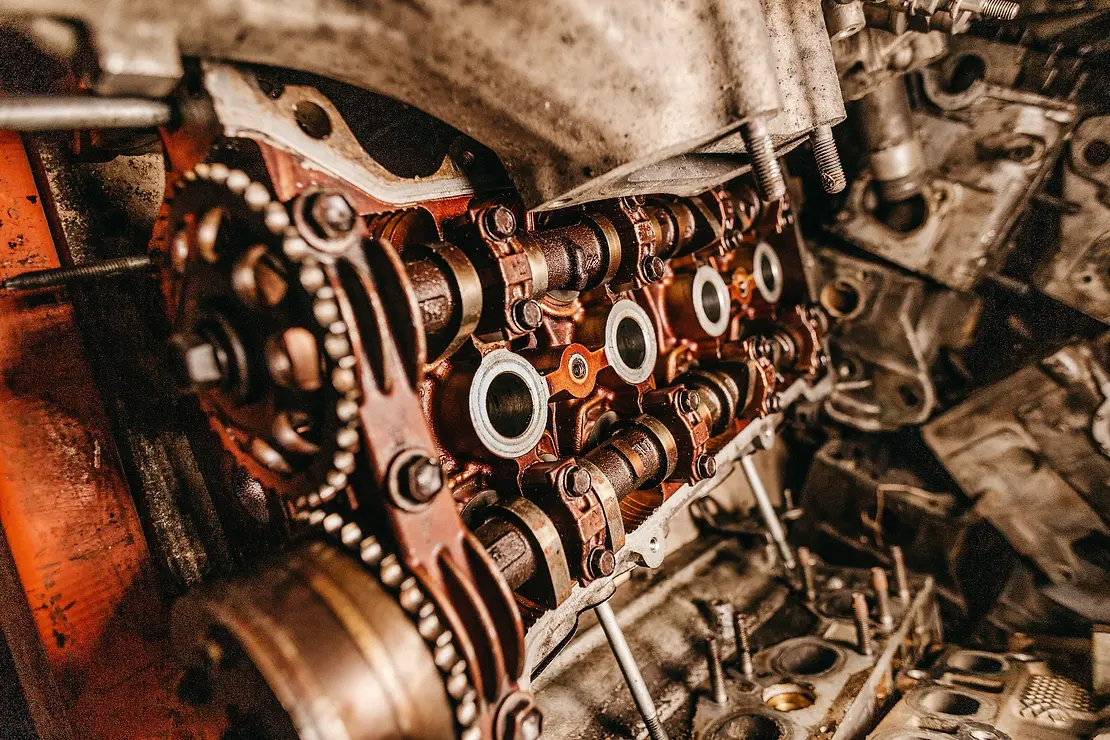
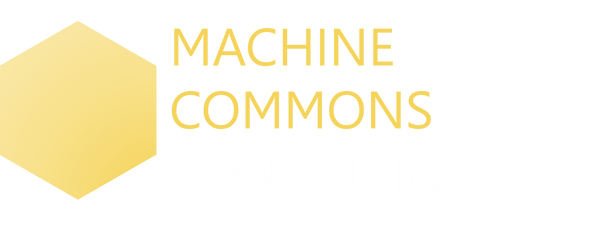
manufacturing
Connect your data environment and optimise processes.
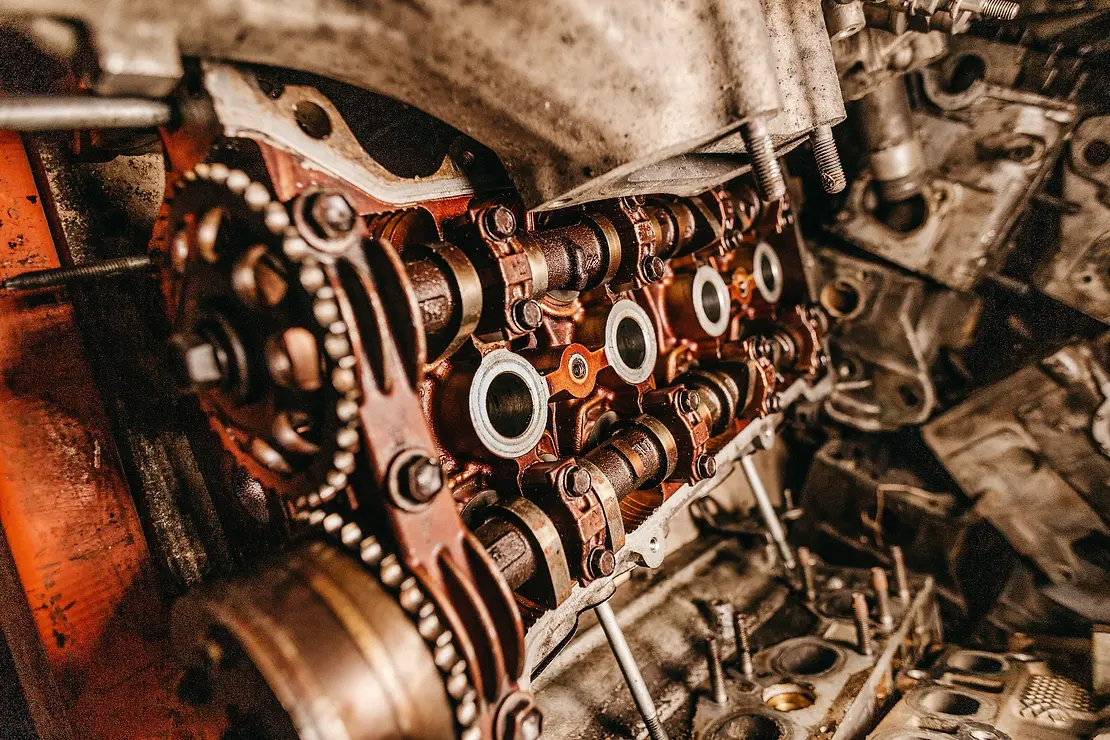
Scale up.
Optimise.
Reduce costs.
Speed up.
Analyse everything.
Of all fields, machine learning is likely to impact manufacturing the most.
Improvements in manufacturing come from an increase in quality, an improvement in speed, a reduction in operating costs or the optimal combination of manufacturing variables.
Machine learning increases what can be automated, can algorithmically determine causal variables of defective production, and can use historical logs to predict undesirable events in the future so they may be avoided.
Optimise production variables like a machine
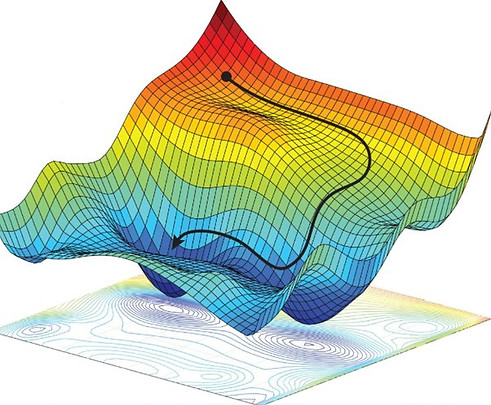
From data to automated optimisations.
Capture everything.
Create an advanced digital environment that ingests all data in real time.
Engage platforms for off the shelf solutions, or enlist engineering firms to build fully customised data environments.
Machine Learning functionality is built on the foundation of a connected data environment. Custom solutions can involve the installation of new sensors that feed any further required data into your 'data historian'.
Analyse everything.
Analyse all variables without needing to understand (or control) all processes.
There exist specialist platforms within the Collective that allow engineers and subject matter experts to rapidly build low/no-code AI models of their industrial equipment, processes or plant operations.
Alternatively, boutique engineering firms can apply hard-won experience to craft fully customised analytical systems built for purpose.
Alternatively,
Optimise everything.
Build, test and iterate countless models against your manufacturing data.
Machine learning models have proven their industry worth beyond measure.
Such as: predicting failures, predicting a given value, identifying the root cause of an event, identifying when equipment is performing sub-optimally or predicting when equipment maintenance is required to avoid downtime.
What do you have to gain?
Live error recognition.
Pattern recognition is perfect for detecting when data streams produce signals they shouldn't be.
Detect defects automatically.
Computer vision systems (or other sensors, such as vibrational) can automatically scan raw materials or production outputs for defects.
Predict values or outcomes.
Foresee yields, energy consumption or qualities from a variable change. Predict seasonal demands or optimal supply chain routes. Determine contributing factors to breakage.
Determine root causes.
Algorithmic approaches can determine which feature variables the most relevant to a search criteria, helping you to arrive at root causes fast - without needing to fully understand underlying processes.
Optimise variable settings.
Machine learning can help answer the complex series of questions associated with production optimisation.
Reduce reliance on humans.
Data automations can reduce human errors, whether it's in simple data migration or with setting inputs. A data-led ecosystem captures everything by default and links every action to a fully tracked causal chain of events.
Reduce machine down time.
Predictive maintenance not only flags when machinery should be maintained. It helps you avoid unnecessary maintenance and therefore reduces machine downtime.
Optimise electricity consumption.
More relevant now than ever, optimising electricity consumption is a crucial cost saver. Malfunctioning equipment or misconfigured systems can be picked up as anomalistic in real time, flagging managing staff.
Scale production with efficiency.
Accurately determining bottle necks, auto-rescheduling resources, maximising production runs and optimising settings directly influence the ability to scale output.
Improve quality control.
Quality can be impacted from the raw material quality, the production process, the supply chain and human handling at any point along this chain. Tracking material to end-product

"this sounds expensive"
Machine learning initiatives directly impact the bottom line by immediately increasing production rates or reducing costs. Therefore every scoped piece of work implies a clear payback period, which tends to be less than 12 months.
Combining machine learning with physics based modelling.
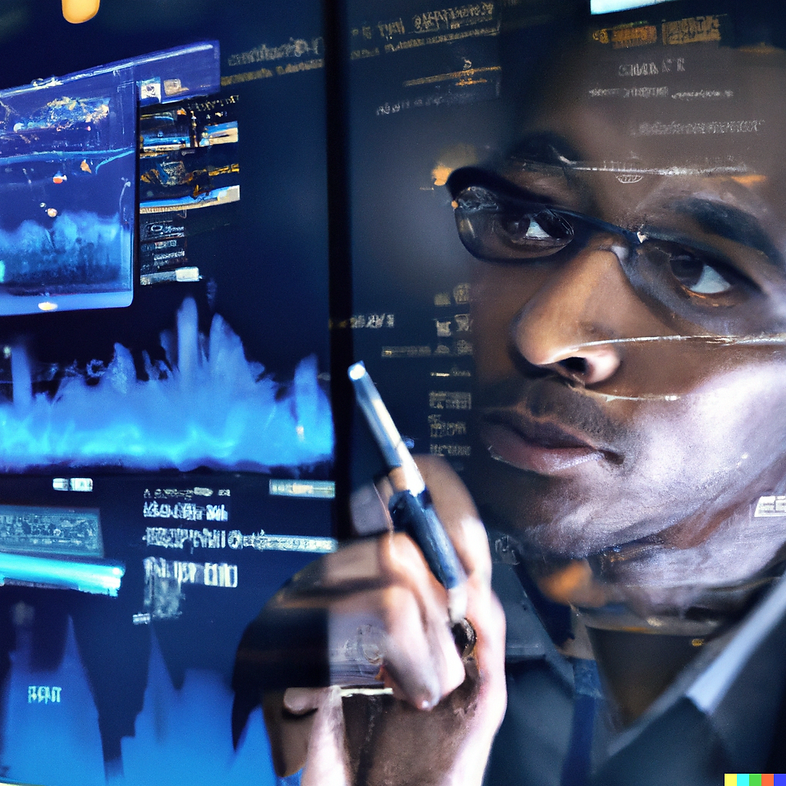
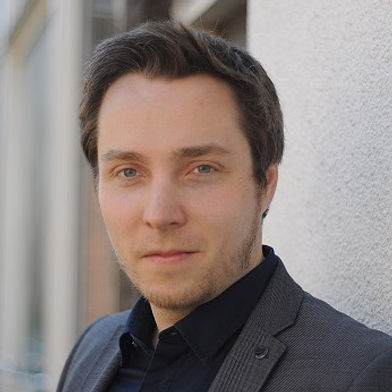
I began to see opportunities that can be solved with machine learning all over the business. Machine Commons answered requests for capability and delivered proposals that I built into my research and presented to C-Suite. Everyone was happy!